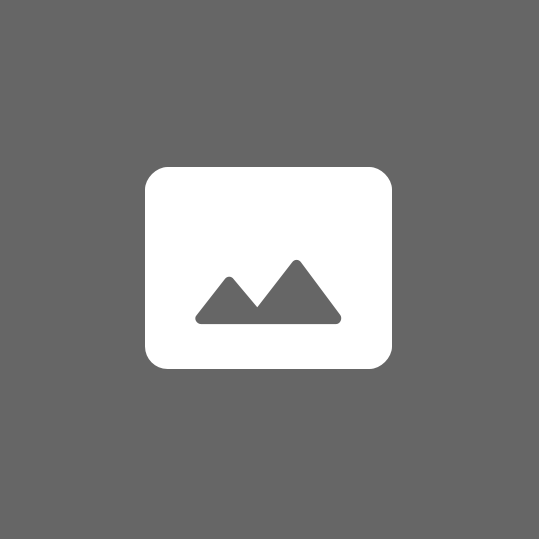
Advertising on the Telegram channel «Data science and Machine Learning»
Quality audience, Educated and English speaking people in the channel who are interested in learning about Data science and Machine Learning.
▪️ Conversion rate is also high (depends on your adds)
▪️ Promotion catogory:-
▪️ Business Adds
▪️ Cryptocurrency Adds
▪️ Tech Adds
▪️ Article Adds
▪️ Educational Adds
▪️ Entartainment Adds
Buy advertising in this channel
- 1/24
- 2/48
- 3/72
- Native
- 7 days
- Forwards
- 1
- 2
- 3
- 4
- 5
- 8
- 10
- 15
Reviews channel
- Added: Newest first
- Added: Oldest first
- Rating: High to low
- Rating: Low to high
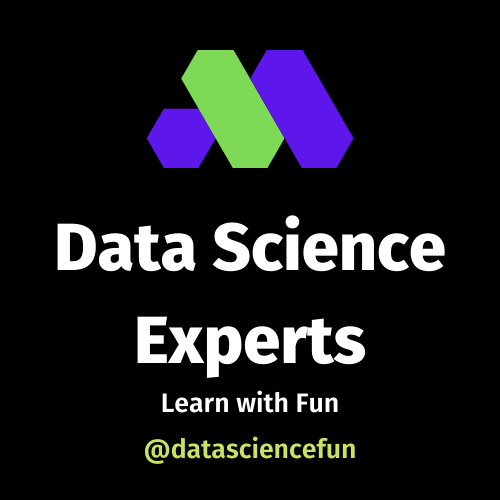
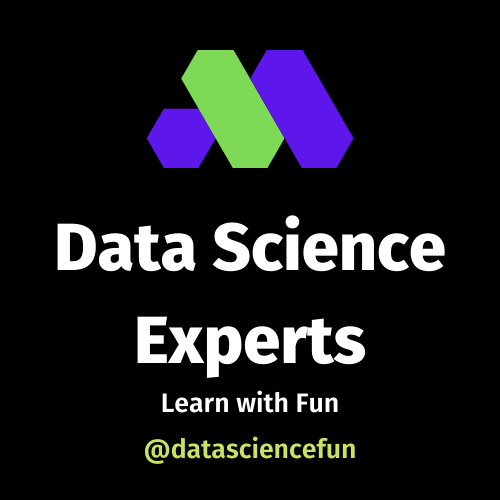
Комментарий