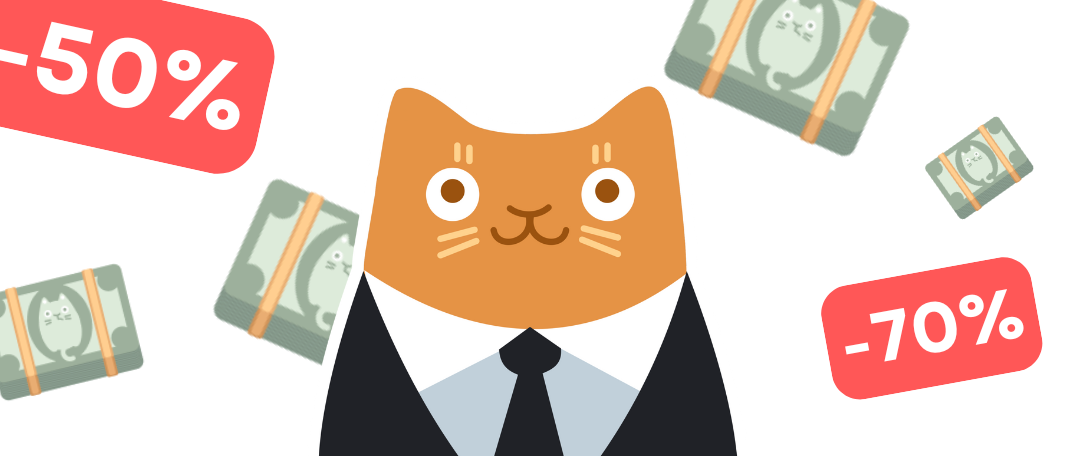
💸 Discounts up to 70% for Business & Finance
Catch the best ad slots in business-related channels — only until April 6!
Grab discount
19.1
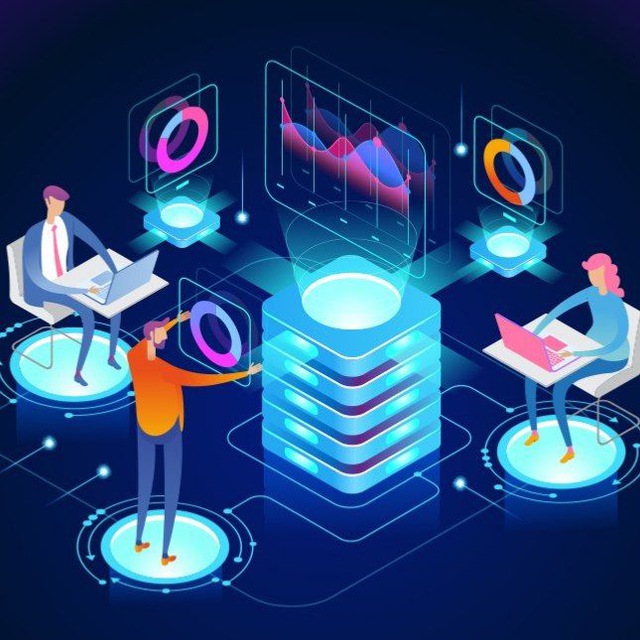
Advertising on the Telegram channel «Data Science | Machine Learning for Researchers»
4.6
33
Computer science
Language:
English
1.4K
3
Share
Add to favorite
Buy advertising in this channel
Placement Format:
keyboard_arrow_down
- 1/24
- 2/48
- 3/72
- Native
- 7 days
- Forwards
1 hour in the top / 24 hours in the feed
Quantity
%keyboard_arrow_down
- 1
- 2
- 3
- 4
- 5
- 8
- 10
- 15
Advertising publication cost
local_activity
$6.00$6.00local_mall
0.0%
Remaining at this price:0
Recent Channel Posts
Explore the world of Data Science through Jupyter Notebooks—insights, tutorials, and tools to boost your data journey. Code, analyze, and visualize smarter with every post.
https://t.me/DataScienceN
81
12:56
04.04.2025
We have launched a support and donation campaign for our channel in order to improve the content and enrich it with more paid resources. You can donate to our channel using stars through this post.
Thanks to everyone who contributes to improving our content, the benefit will be extended to all members
156
11:18
04.04.2025
imageImage preview is unavailable
Effect-driven interpretation: Functors for natural language composition
🖥 Github: https://github.com/UCSC-VLAA/MedReason
📕 Paper: https://arxiv.org/abs/2504.00993v1
🔗 Tasks: https://paperswithcode.com/task/knowledge-graphs
307
11:17
04.04.2025
imageImage preview is unavailable
Open Deep Search: Democratizing Search with Open-source Reasoning Agents
26 Mar 2025 · Salaheddin Alzubi, Creston Brooks, Purva Chiniya, Edoardo Contente, Chiara von Gerlach, Lucas Irwin, Yihan Jiang, Arda Kaz, Windsor Nguyen, Sewoong Oh, Himanshu Tyagi, Pramod Viswanath ·
We introduce Open Deep Search (ODS) to close the increasing gap between the proprietary search AI solutions, such as Perplexity's Sonar Reasoning Pro and OpenAI's GPT-4o Search Preview, and their open-source counterparts. The main innovation introduced in ODS is to augment the reasoning capabilities of the latest open-source LLMs with reasoning agents that can judiciously use web search tools to answer queries. Concretely, ODS consists of two components that work with a base LLM chosen by the user: Open Search Tool and Open Reasoning Agent. Open Reasoning Agent interprets the given task and completes it by orchestrating a sequence of actions that includes calling tools, one of which is the Open Search Tool. Open Search Tool is a novel web search tool that outperforms proprietary counterparts. Together with powerful open-source reasoning LLMs, such as DeepSeek-R1, ODS nearly matches and sometimes surpasses the existing state-of-the-art baselines on two benchmarks: SimpleQA and FRAMES. For example, on the FRAMES evaluation benchmark, ODS improves the best existing baseline of the recently released GPT-4o Search Preview by 9.7% in accuracy. ODS is a general framework for seamlessly augmenting any LLMs -- for example, DeepSeek-R1 that achieves 82.4% on SimpleQA and 30.1% on FRAMES -- with search and reasoning capabilities to achieve state-of-the-art performance: 88.3% on SimpleQA and 75.3% on FRAMES.Paper: https://arxiv.org/pdf/2503.20201v1.pdf Code: https://github.com/sentient-agi/opendeepsearch
#DataScience #ArtificialIntelligence #MachineLearning #PythonProgramming #DeepLearning #LLM #AIResearch #BigData #NeuralNetworks #DataAnalytics #NLP #AutoML #DataVisualization #ScikitLearn #Pandas #NumPy #TensorFlow #AIethics #PredictiveModeling #GPUComputing #OpenSourceAI #DeepSeek #RAG #Agents #GPT4 https://t.me/DataScienceT
455
07:05
04.04.2025
imageImage preview is unavailable
🙏💸 500$ FOR THE FIRST 500 WHO JOIN THE CHANNEL! 🙏💸
Join our channel today for free! Tomorrow it will cost 500$!
https://t.me/+vhF2zNz5GBw3NTU1
You can join at this link! 👆👇
https://t.me/+vhF2zNz5GBw3NTU1
321
12:09
03.04.2025
imageImage preview is unavailable
This channels is for Programmers, Coders, Software Engineers.
0️⃣ Python
1️⃣ Data Science
2️⃣ Machine Learning
3️⃣ Data Visualization
4️⃣ Artificial Intelligence
5️⃣ Data Analysis
6️⃣ Statistics
7️⃣ Deep Learning
8️⃣ programming Languages
✅ https://t.me/addlist/8_rRW2scgfRhOTc0
✅ https://t.me/Codeprogrammer
372
20:54
02.04.2025
imageImage preview is unavailable
Harnessing the Reasoning Economy: A Survey of Efficient Reasoning for Large Language Models
🖥 Github: https://github.com/devoallen/awesome-reasoning-economy-papers
📕 Paper: https://arxiv.org/abs/2503.24377v1
807
19:59
02.04.2025
imageImage preview is unavailable
Long-Context Autoregressive Video Modeling with Next-Frame Prediction
25 Mar 2025 · YuChao Gu, Weijia Mao, Mike Zheng Shou ·
Long-context autoregressive modeling has significantly advanced language generation, but video generation still struggles to fully utilize extended temporal contexts. To investigate long-context video modeling, we introduce Frame AutoRegressive (FAR), a strong baseline for video autoregressive modeling. Just as language models learn causal dependencies between tokens (i.e., Token AR), FAR models temporal causal dependencies between continuous frames, achieving better convergence than Token AR and video diffusion transformers. Building on FAR, we observe that long-context vision modeling faces challenges due to visual redundancy. Existing RoPE lacks effective temporal decay for remote context and fails to extrapolate well to long video sequences. Additionally, training on long videos is computationally expensive, as vision tokens grow much faster than language tokens. To tackle these issues, we propose balancing locality and long-range dependency. We introduce FlexRoPE, an test-time technique that adds flexible temporal decay to RoPE, enabling extrapolation to 16x longer vision contexts. Furthermore, we propose long short-term context modeling, where a high-resolution short-term context window ensures fine-grained temporal consistency, while an unlimited long-term context window encodes long-range information using fewer tokens. With this approach, we can train on long video sequences with a manageable token context length. We demonstrate that FAR achieves state-of-the-art performance in both short- and long-video generation, providing a simple yet effective baseline for video autoregressive modeling.Paper: https://arxiv.org/pdf/2503.19325v1.pdf Code: https://github.com/showlab/FAR Dataset: UCF101 Ranked #2 on Video Generation on UCF-101 https://t.me/DataScienceT ⚠️
1199
14:28
01.04.2025
imageImage preview is unavailable
LHM: Large Animatable Human Reconstruction Model from a Single Image in Seconds
13 Mar 2025 · Lingteng Qiu, Xiaodong Gu, Peihao Li, Qi Zuo, Weichao Shen, Junfei Zhang, Kejie Qiu, Weihao Yuan, GuanYing Chen, Zilong Dong, Liefeng Bo ·
Animatable 3D human reconstruction from a single image is a challenging problem due to the ambiguity in decoupling geometry, appearance, and deformation. Recent advances in 3D human reconstruction mainly focus on static human modeling, and the reliance of using synthetic 3D scans for training limits their generalization ability. Conversely, optimization-based video methods achieve higher fidelity but demand controlled capture conditions and computationally intensive refinement processes. Motivated by the emergence of large reconstruction models for efficient static reconstruction, we propose LHM (Large Animatable Human Reconstruction Model) to infer high-fidelity avatars represented as 3D Gaussian splatting in a feed-forward pass. Our model leverages a multimodal transformer architecture to effectively encode the human body positional features and image features with attention mechanism, enabling detailed preservation of clothing geometry and texture. To further boost the face identity preservation and fine detail recovery, we propose a head feature pyramid encoding scheme to aggregate multi-scale features of the head regions. Extensive experiments demonstrate that our LHM generates plausible animatable human in seconds without post-processing for face and hands, outperforming existing methods in both reconstruction accuracy and generalization ability.Paper: https://arxiv.org/pdf/2503.10625v1.pdf Code: https://github.com/aigc3d/LHM https://t.me/DataScienceT ⚠️
1444
14:24
31.03.2025
imageImage preview is unavailable
InfiniteYou: Flexible Photo Recrafting While Preserving Your Identity
20 Mar 2025 · Liming Jiang, Qing Yan, Yumin Jia, Zichuan Liu, Hao Kang, Xin Lu ·
Achieving flexible and high-fidelity identity-preserved image generation remains formidable, particularly with advanced Diffusion Transformers (DiTs) like FLUX. We introduce InfiniteYou (InfU), one of the earliest robust frameworks leveraging DiTs for this task. InfU addresses significant issues of existing methods, such as insufficient identity similarity, poor text-image alignment, and low generation quality and aesthetics. Central to InfU is InfuseNet, a component that injects identity features into the DiT base model via residual connections, enhancing identity similarity while maintaining generation capabilities. A multi-stage training strategy, including pretraining and supervised fine-tuning (SFT) with synthetic single-person-multiple-sample (SPMS) data, further improves text-image alignment, ameliorates image quality, and alleviates face copy-pasting. Extensive experiments demonstrate that InfU achieves state-of-the-art performance, surpassing existing baselines. In addition, the plug-and-play design of InfU ensures compatibility with various existing methods, offering a valuable contribution to the broader community.Paper: https://arxiv.org/pdf/2503.16418v1.pdf Code: https://github.com/bytedance/infiniteyou Dataset: 10,000 People - Human Pose Recognition Data https://t.me/DataScienceT ⚠️
1628
14:11
30.03.2025
close
Reviews channel
keyboard_arrow_down
- Added: Newest first
- Added: Oldest first
- Rating: High to low
- Rating: Low to high
4.6
1 reviews over 6 months
Very good (100%) In the last 6 months
e
**ikpelli@********.com
On the service since January 2025
07.01.202516:57
4
ad has been placed
Show more
New items
Channel statistics
Rating
19.1
Rating reviews
4.6
Сhannel Rating
82
Followers:
28.3K
APV
lock_outline
ER
2.3%
Posts per day:
0.0
CPM
lock_outlineSelected
0
channels for:$0.00
Followers:
0
Views:
lock_outline
Add to CartBuy for:$0.00
Комментарий